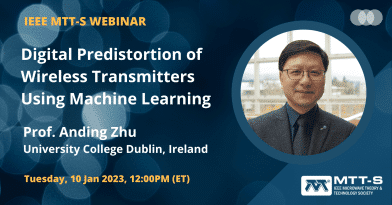
-
Tuesday, January 10, 2023 12:00 pm - 1:00 pm(New York Time) Add to my calendar
Digital Predistortion of Wireless Transmitters Using Machine Learning
Prof. Anding Zhu
University College Dublin, Ireland
Abstract: Digital predistortion (DPD) has been widely adopted to keep RF power amplifier operating with high efficiency without losing linearity in the exiting 4G systems. It is expected that DPD will continue to be deployed in 5G systems. However, due to shifting from the single antenna to the multiple-input multiple-output (MIMO) phased array and continuously increased signal bandwidth, system designers face significant challenges in managing power consumption and meeting linearity requirement of wireless transmitters. In this talk, we will discuss the recent advances in DPD development that can resolve some of the issues in linearizing 5G MIMO systems using machine learning, including DPD system architectures, model order reduction, fast model adaptation and power reduction techniques.
Speaker’s Bio: Anding Zhu received the Ph.D. degree in electronic engineering from University College Dublin (UCD), Dublin, Ireland, in 2004. He is currently a Professor with the School of Electrical and Electronic Engineering at UCD. His research interests include high-frequency nonlinear system modeling and device characterization techniques, high-efficiency power amplifier design, wireless transmitter architectures, and nonlinear system identification algorithms. He has published more than 200 peer-reviewed journal and conference articles. Prof. Zhu is an IEEE Fellow. He served as the Secretary of Administrative Committee (AdCom) of IEEE Microwave Theory and Technology Society (MTT-S) in 2018. He has been an Elected Member of MTT-S AdCom since 2019 and a Member of IEEE Future Directions Committee since 2000. Prof. Zhu served as a Track Editor of IEEE Transactions on Microwave Theory and Techniques in 2020-2022 and he was a recipient of 2021 IEEE MTT-S Microwave Prize.